Early warnings and predictive maintenance on critical assets
Use case
Challenge
Equipment can break or reduce in efficiency over time. Machine learning algorithms can learn how equipment is supposed to operate under various modes and seasons. It can then warn maintenance engineers when an asset or process is not behaving as expected. However, this requires data science competency and often rather long and complex implementation projects.
Solution
With Intelecy, the maintenance engineers build and deploy these predictive models themselves in a few simple clicks, making it possible to monitor hundreds of assets without any involvement from specialists, such as data scientists. The results are fewer failures, outages, and ad-hoc maintenance jobs. A “simple” example can be that the temperature in circuit breakers or transformers is often monitored with static alarm limits. A static alarm limit doesn’t consider ambient temperature and the load.
Intelecy models will have dynamic alarm thresholds based on multiple variables, so 60 degrees in the summer may be ok, but not in the winter. This limits false alarms and ensures operators can take actions based actual events.
Result
- Reduced emissions
- Early warnings provided
- Enhanced process efficiency
Other Power & Renewable Energy use cases
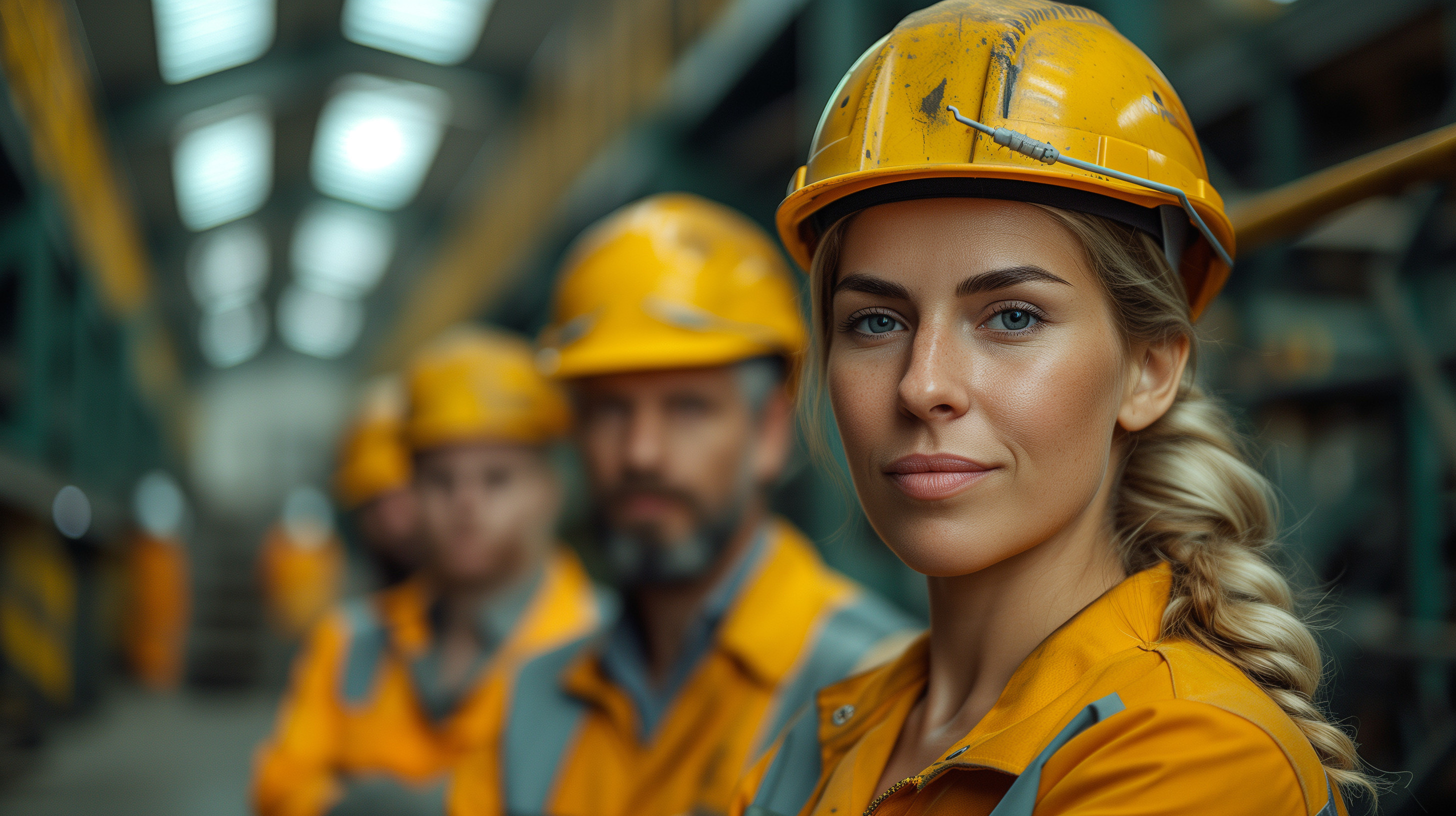
Actionable insights to reduce emissions
Discover how no-code AI models can provide actionable insights for emission reduction by predicting the future value of harmful gases and providing early warnings of deviations in the cleaning process.
.jpeg?width=4741&height=3314&name=AdobeStock_219968252%20(1).jpeg)
Forecast the future of energy demands
By producing in an optimal balance between supply and demand, the energy plant will improve raw material utilization and increase production and distribution cost efficiency. Discover how forecast no-code machine learning models predict the future energy demand.