Why should the process industry care about AI?
As I wrote about in my last post, the process industry is facing challenges that are impacting its growth and profitability. These challenges include rising energy costs, increased competition, strict regulatory compliance requirements and a retiring workforce. In this context, organizations must find ways to optimize their processes and improve efficiency to stay competitive. A step-change is needed!
AI - a game changer for manufacturers
ChatGPT and OpenAI are making headlines. The recent “hype” around AI has generated significant interest in its applications within the process industry, and more and more organizations are starting to realize that AI could be a game changer when it comes to solving industrial challenges.
Before diving into the opportunities, are you still wondering what the difference is between AI, Machine Learning, and statistics? You’re not alone! This blog post “Demystifying AI & ML in Industrial Analytics” by Joe Perino, Research Analyst with LNS Research, is a great place to start.
-
Process Optimization: AI can optimize complex manufacturing processes by analyzing data from sensors, production lines, and other sources like lab tests. This will enable operators and process engineers to identify bottlenecks and make process adjustments to improve efficiency and overall productivity.
-
Quality Control: AI can analyze vast amounts of data to detect defects or anomalies in the manufacturing process. AI can monitor sub-processes in real time and enable operators to make process adjustments or stop production, preventing the production of products that will not meet quality standards. This can have a significant impact on reduced costs and waste.
-
Energy Management: Forecasting short-term energy demand is challenging. Operators have a fairly good understanding of normal daily and weekly variations; however, it´s more complicated to understand if the next hour will have higher or lower demand than “normal.” AI can monitor energy consumption patterns in real time. Identifying opportunities for energy conservation and efficiency improvements can contribute to cost savings and environmental sustainability.
-
Predictive Maintenance: Perhaps the most known type of use case: AI can analyze sensor data from machinery and equipment to identify patterns and indicators of potential failures. By predicting maintenance needs in advance, companies can avoid unplanned downtime, reduce maintenance costs, and optimize the use of resources.
-
Closing the Knowledge Gap: As experienced workers retire, they take with them industry-specific knowledge, best practices, and insights. By analyzing historical and real-time data, AI applications can assist less-experienced workers in making informed and data-driven decisions. These tools can also help attract new talent, as the younger and more tech-savvy generation will have expectations when it comes to modern data-driven technologies.
Realizing ROI from AI projects remains a challenge
The opportunity exists; however, according to Gartner 85% of all AI projects fail to meet business objectives. Worse yet, the research company predicts that this trend will continue. Other research shows that 93% of Organizations Committed to AI but Skills Shortage Poses Considerable Challenge. The key reasons highlighted as reason for failure are the lack of access to:
- skilled talent
-
user-friendly technology/tools
-
industrial data
This corresponds well with what we are hearing in client dialogues.
There is a shortage of professionals who can build effective AI solutions. Many industrial organizations are tied back by not having the right in-house competence and struggle with, or have no interest in, hiring internal data scientists. Engaging external consultants is a high risk of failure as they often do not have adequate knowledge about the specific industrial processes.
Accessing Operational Technology (OT) data is often more challenging than accessing Information Technology (IT) data due to legacy systems, security concerns, and the diverse data types involved. OT systems often lack standardization and require real-time access, and regulatory compliance further complicates data retrieval. Bridging the IT-OT gap requires expertise in both domains and efforts to overcome vendor dependencies and organizational barriers.
The benefit of no-code AI software software lies in empowering employees without technical and programming expertise to create and use Machine Learning models, and hence harness the power of AI. In addition, you do not have to invest in complex, expensive, and hard-to-scale “Do-it-yourself” (DIY) projects.
Let’s look at some more specific advantages:
-
Empowerment of domain experts: By leveraging no-code AI solutions, domain experts can be the driving force in AI initiatives. Their expertise understanding of the industrial challenges and contextual knowledge make them a key to success. They can actively build and fine-tune Machine Learning models, resulting in solutions more aligned with the specific needs and nuances of the process industry.
-
Wider organizational adoption: No-code AI software democratizes AI capabilities within the organization. This broader accessibility fosters a culture of innovation and encourages a wider range of employees to contribute to solving challenges by leveraging AI.
-
Rapid prototyping and experimentation: No-code AI tools allow users to test and validate ideas and use cases quickly. Process engineers can easily create and modify Machine Learning models and assess their impact on performance. This iterative approach enables the identification of optimal solutions and reduces the risk associated with implementing complex AI projects.
-
Faster time to ROI: While IT-driven “DIY” AI projects can generate great results, they often face scalability challenges compared to purchasing Software-as-a-Service (SaaS) no-code AI software. Building and maintaining your own data integrations and AI solutions require significant time, resources, and expertise, which might not be scalable as your needs grow.
Risk of getting left behind - get started today!
A recent forecast from IDC shows that Worldwide Spending on AI-Centric Systems Forecast to Reach $154 Billion in 2023 ****and increase by 27% from 2022 to 2023. If counting manufacturing as the sum of discrete manufacturing and process manufacturing, then the manufacturing industry will be the top industry regarding AI investments. "Companies that are slow to adopt AI will be left behind – large and small," says Mike Glennon, senior market research analyst with IDC's Customer Insights & Analysis team.
The Intelecy platform also solves the access to OT data issue, as the solution was built for industrial data, and securely and swiftly integrates with standard industrial protocols and systems. This ensures fast onboarding and ultra-scalable deployment across sites. The Intelecy Gateway enables a fast and secure flow of data between your industrial data sources, like historian or distributed control system (DCS), and the Intelecy platform.
Explore how process industry organizations are leveraging Intelecy to achieve substantial business value. Please reach out if you’d like a tailored demo or have any questions. We’re here to help you kick-start and succeed in your AI initiatives!
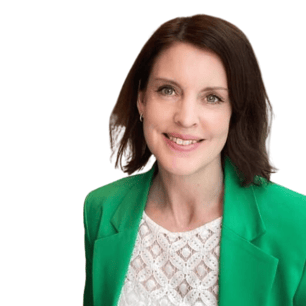