How AI is revolutionizing the chemical industry: Process optimization, energy management, and predictive maintenance
The chemical industry, once dominated by manual processes and rigid systems, is now experiencing a technological revolution. Artificial intelligence (AI) is no longer a futuristic concept but a real-time, transformative tool that is optimizing operations, reducing energy consumption, and driving sustainable innovation. And it’s happening faster than ever.
Chemical companies utilize AI to optimize production processes.
With AI, chemical companies are not only optimizing processes and enhancing safety—they are unlocking potential for growth and innovation in ways that were previously unimaginable. Let’s dive into the specific challenges faced by the industry and how AI is offering unprecedented solutions.
Real-time Process Optimization with AI
Challenge:
In the fast-paced world of chemical production, process optimization is both the biggest opportunity and the hardest challenge. Achieving optimal efficiency in chemical processes is complex due to numerous variables. These processes often involve high temperatures, pressures, flow-rates and the precise control of chemical reactions, making manual optimization difficult and time-consuming.
Solution:
AI models can analyze real-time data to optimize process parameters. By integrating data from sensors and control systems, AI models provide predictions that allow operators to fine-tune processes, enhancing efficiency and product quality. AI is not just improving these processes—it’s enabling making real-time, intelligent decisions that would be impossible for humans to replicate.
Use case: Process optimization and yield forecasting
Distillation columns are key in separating chemical mixtures. An AI model can optimize the operation of these columns by adjusting parameters such as reflux ratio, feed temperature, and pressure. AI can also forecast product purity and yield based on operating conditions.
In this case a Machine Learning model predicts that a slight increase in reflux ratio and adjustment of feed preheat temperature will improve benzene purity by 0,5% without increasing energy consumption. The system continuously adjusts these variables to maintain optimal performance.
Use case: Temperature control and product consistency
Forehearths are used to condition molten glass before it reaches the forming equipment. The use of AI can help control the temperature profile along the forehearth to ensure uniform viscosity and prevent defects in the glass.
In this case a Machine Learning model monitors the temperature along a forehearth and adjusts heating zones dynamically to maintain a uniform viscosity in the molten glass. This reduces product defects such as bubbles and inclusions by 3% and ensures higher-quality glass products.
Energy management optimization
Challenge:
Chemical processes such as distillation and electrolysis consume large amounts of energy, leading to high operational costs and a significant environmental footprint. Efficient energy management is crucial to reducing both expenses and emissions.
Solution:
AI can optimize energy usage by analyzing consumption patterns and predicting where energy savings can be made. By making adjustments to operations without compromising output, companies can cut costs while improving sustainability.
Use case: Fouling detection and energy optimization
Heat exchangers are prone to fouling (build-up of deposits), which reduces their efficiency over time. AI models can predict fouling by analyzing inlet/outlet temperatures, flow rates, and pressure drops, and optimize cleaning schedules to prevent energy loss. In one example, a Machine Learning model predicts when fouling in a heat exchanger will reach a critical point, allowing cleaning to be scheduled just in time. This reduces energy consumption by 4% while maintaining performance.
Use case: Optimization of water usage and energy efficiency
Cooling towers are essential for dissipating heat in chemical plants. AI models can optimize cooling tower operations by analyzing water temperature, flow rates, and ambient conditions, reducing energy consumption and water usage. In this case Machine Learning model monitors a boiler’s fuel consumption and identifies a decrease in efficiency due to fouling in the heat exchanger. It recommends cleaning the heat exchanger to restore optimal efficiency, saving fuel costs and reducing CO₂ emissions by 5%.
Waste reduction and sustainability
Challenge:
Waste reduction and sustainability are crucial for the chemical industry, where efficient resource utilization is paramount. Minimizing waste allows companies to reduce costs and optimize operations, and meet stringent environmental regulations.
Solution:
AI can optimize resource utilization, reduce waste generation, and improve recycling processes. Machine learning models identify inefficiencies and suggest more sustainable practices. By cutting pollution and conserving natural resources, businesses can not only improve their bottom line but also establish themselves as leaders in sustainability.
Use case: Emission monitoring and filter blockage detection
Scrubbers and filters are used for removing contaminants from gas streams. AI models can optimize scrubbing efficiency by adjusting operating conditions and predicting when filters are nearing capacity, helping avoid blockages that could lead to operational inefficiency. In this case a a Machine Learning model monitoring a wet scrubber detected fluctuations in outlet gas composition, signaling inefficient contaminant removal. By adjusting pH levels in the scrubbing liquid, the system restored scrubber efficiency, while predicting filter clogging early to avoid operational inefficiencies.
Use case: Emission monitoring and reduced emissions
Boilers used to generate steam in chemical processes can also be optimized with AI. Machine Learning models analyze fuel consumption, steam output, and emissions, identifying issues such as burner fouling or heat exchanger scaling. In this case, a model monitoring boiler performance predicted decreased efficiency due to fouling in the heat exchanger, recommending cleaning, which reduced CO₂ emissions by 5%.
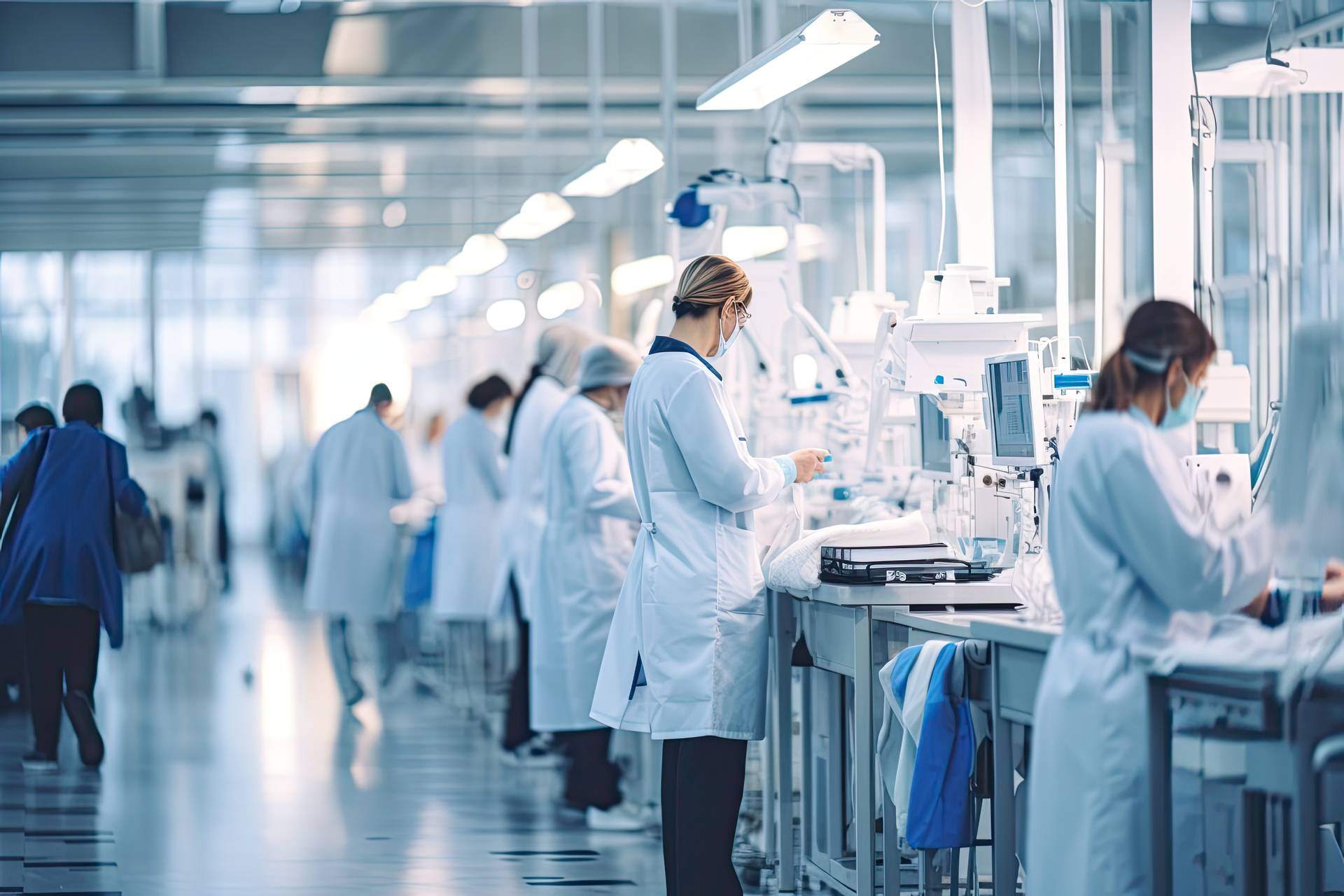
Engineers in the chemical industry drive safety and innovation with no-code AI
Predictive maintenance
Challenge:
Unplanned downtime of critical equipment such as chemical reactors, distillation columns, and heat exchangers can lead to significant financial losses and production delays. Traditional maintenance approaches, based on fixed schedules or reactive responses to equipment failure, often result in either over-maintenance or unexpected breakdowns.
Solution:
Predictive maintenance is a key opportunity for the chemical industry, enabling companies to anticipate equipment failures before they occur. AI algorithms can predict equipment failures by analyzing historical data, sensor readings, and operational conditions. Machine learning models analyze this data to identify patterns and anomalies that precede equipment failures, preventing costly breakdowns, reducing repair expenses, and extending equipment lifespan.
Use case: Predictive Maintenance for pump failures
Centrifugal pumps are vital for transferring liquids in chemical plants. AI models can monitor vibration, pressure, and temperature data from sensors, detecting early signs of issues like bearing wear or impeller damage. In this case, a Machine Learning model detected an abnormal spike in vibration frequencies, indicating early stages of impeller wear. It predicted a failure within a week, allowing maintenance staff to replace the pump before an unplanned shutdown occurred.
Use case: Anomaly detection and energy efficiency
Compressors used in chemical processes benefit from AI-powered anomaly detection. By monitoring performance metrics like discharge pressure and energy consumption, AI models can detect potential failures and optimize energy usage. In this case, a Machine Learning model monitoring a reciprocating compressor in an ammonia synthesis plant identified a valve malfunction by detecting an abnormal increase in discharge temperature, triggering early maintenance and reducing downtime.
Implementation considerations
Data Integration
Successful AI implementation requires integrating data from various sources, including sensors, historical records, and external databases. Ensuring data quality and consistency is crucial for accurate analysis and reliable AI models.
Skill Development
Workforce training in AI and data analytics are crucial for the effective adoption and utilization of AI technologies in the chemical industry. The scarcity of data scientists and the growing number of experienced employees nearing retirement pose significant challenges. Chemical companies must not only invest in upskilling their current workforce but also address the need to attract and retain new talents in a competitive market.
Scalability
AI solutions should be scalable to accommodate the growing data and evolving needs of the industry. Scalable AI systems can handle increasing data volumes and complexity, ensuring long-term viability and performance.
Collaboration
Collaboration between AI experts, chemical engineers, and domain specialists is essential to tailor AI solutions to specific operational challenges. Cross-disciplinary teams can leverage their combined expertise to develop and implement effective AI strategies.
How Intelecy can help
Data integration
Intelecy’s platform seamlessly integrates data from various sources, including IoT sensors, historical databases, and external systems, creating a unified data environment for comprehensive analysis. Unlike traditional AI solutions that can take months to deploy, Intelecy’s advanced integration capabilities and out-of-the-box data connectors allow you to deploy AI models within weeks. This rapid deployment ensures that businesses can quickly harness the power of AI to optimize processes and improve decision-making without long delays. With Intelecy, chemical companies can swiftly move from data collection to actionable insights, enabling faster time-to-value.
Skill Development
Intelecy’s user-friendly tools and interfaces empower chemical engineers and operators to leverage AI without needing deep data science expertise, helping to bridge the skill gap. Additionally, Intelecy offers training and support to ensure swift onboarding, both when it comes to adoption and identifying use cases. This enables businesses to stay competitive and future-proof their operations despite workforce challenges.
Scalability
Intelecy’s platform is designed to scale with your operations, ensuring that AI capabilities grow as your data and operational needs expand. In addition to supporting large-scale data processing, Intelecy enables the rapid building and operationalizing of AI models, allowing chemical companies to deploy solutions faster and more efficiently. This agility ensures that AI-driven insights can be applied in real-time, leading to immediate improvements in performance, productivity, and innovation. Whether your business is in the early stages of AI adoption or looking to enhance existing systems, Intelecy’s scalable architecture ensures consistent performance and reliability at every stage.
Collaboration
Intelecy facilitates collaboration by providing a common platform where AI experts, chemical engineers, and domain specialists can work together. The platform’s intuitive design allows all stakeholders to contribute and benefit from the AI solutions effectively in one single use-friendly user interface. Intelecy’s collaborative tools support knowledge sharing and collective problem-solving, driving innovation and continuous improvement.
Conclusion
AI is transforming the chemical industry, addressing operational challenges such as predictive maintenance, process optimization, and sustainability. By focusing on key implementation considerations and leveraging a no-code industrial AI, chemical companies can stay competitive and achieve sustainable growth.
By leveraging Intelecy’s capabilities, the chemical industry can address operational challenges more efficiently, enhancing productivity, reducing costs, improving safety, and fostering innovation.
The chemical industry is on the verge of a major transformation, and those who fail to adopt AI risk being left behind. Platforms like Intelecy are leading this revolution, offering accessible, scalable AI solutions tailored to your unique operational needs. The future of chemical production isn’t just smarter—it’s here.
Want to see how AI can take your operations to the next level? Reach out to today and start optimizing for a smarter, more sustainable future - or watch one of our on-demand webinars, to hear how to get started and also hear how our customers are using AI in everyday operations!
GET IN TOUCH